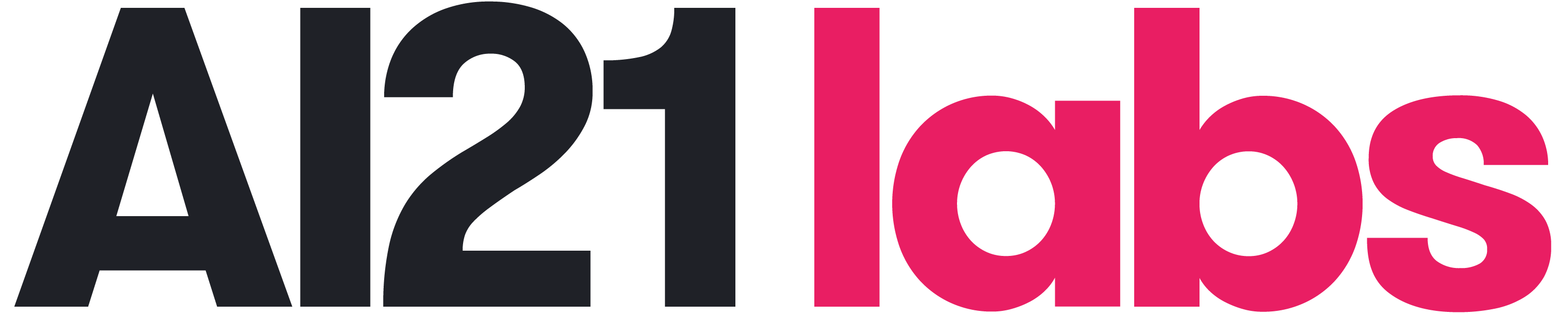
AI21 Labs
Founded Year
2017Stage
Series C - II | AliveTotal Raised
$317MValuation
$0000Last Raised
$53M | 1 yr agoRevenue
$0000About AI21 Labs
AI21 Labs operates as an artificial intelligence (AI) lab and product company. The company offers a range of AI-powered tools, including a writing companion tool to assist users in rephrasing their writing and an AI reader that summarizes long documents. It also provides language models for developers to create AI-powered applications. It was founded in 2017 and is based in Tel Aviv, Israel.
Loading...
AI21 Labs's Product Videos
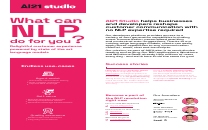
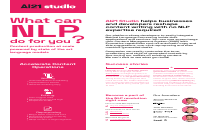
ESPs containing AI21 Labs
The ESP matrix leverages data and analyst insight to identify and rank leading companies in a given technology landscape.
The generative AI — large language model (LLM) developers market offers foundation models and APIs that enable enterprises to build natural language processing applications for a number of functions. These include content creation, summarization, classification, chat, sentiment analysis, and more. Companies in this market develop and train their own large-scale language models — which are pre-trai…
AI21 Labs named as Challenger among 15 other companies, including Google, IBM, and Meta.
AI21 Labs's Products & Differentiators
Jamba 1.6
the most advanced open model for private enterprise deployment, designed to meet real-world business needs without sacrificing security or performance. Built on AI21’s hybrid SSM-Transformer architecture, Jamba 1.6 delivers industry-leading results, outperforming leading models across key enterprise use cases while providing the best accuracy, speed, and security in the market. Jamba 1.6 outperforms its open model competitors from Mistral, Meta, and Cohere across multiple benchmarks, showcasing superior general quality (Arena Hard) and best-in-class performance on retrieval-augmented generation (RAG) and long-context question answering (QA). Critically, Jamba 1.6 achieves these results without compromising on speed, as demonstrated in scatter plot benchmarks.
Loading...
Research containing AI21 Labs
Get data-driven expert analysis from the CB Insights Intelligence Unit.
CB Insights Intelligence Analysts have mentioned AI21 Labs in 8 CB Insights research briefs, most recently on Jan 8, 2025.
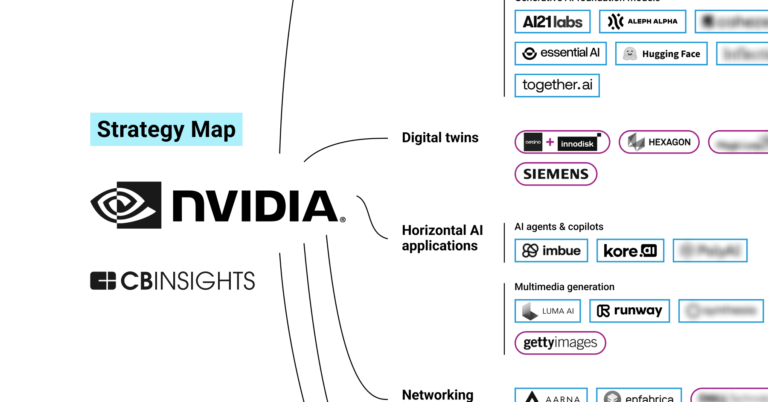
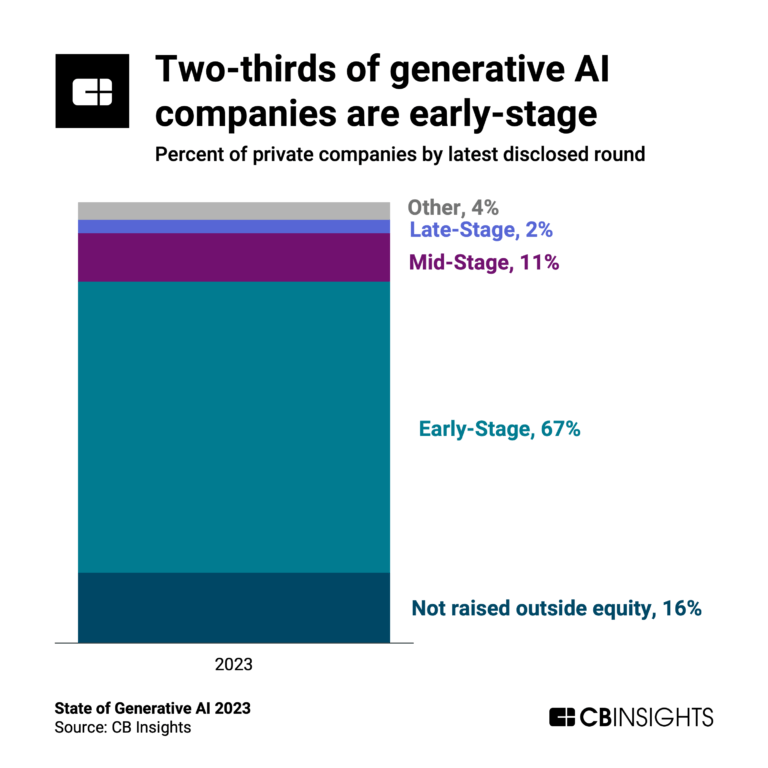
Feb 27, 2024
The generative AI boom in 6 charts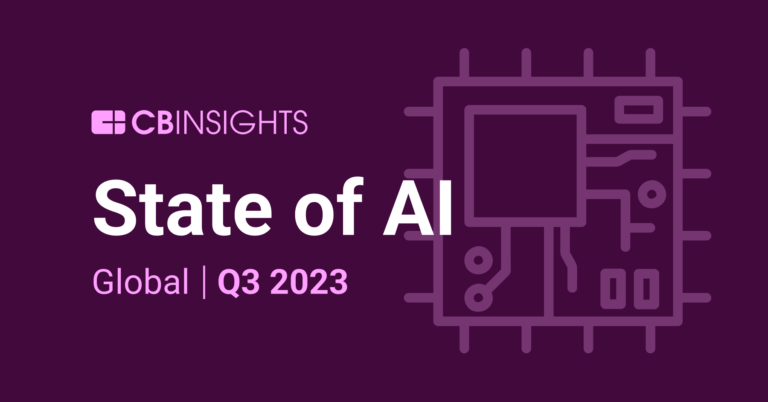
Nov 15, 2023 report
State of AI Q3’23 Report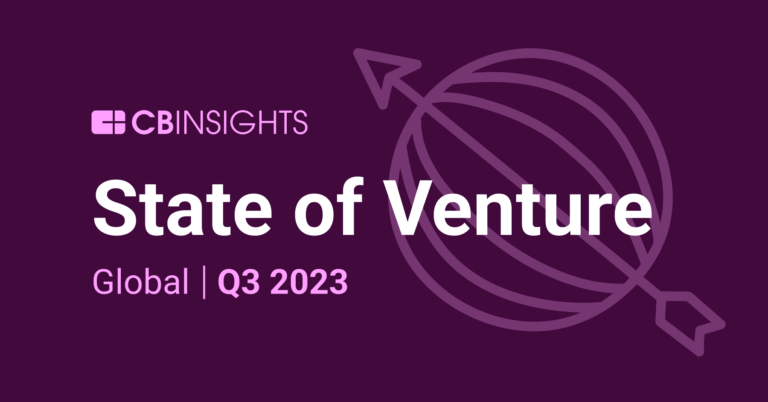
Oct 12, 2023 report
State of Venture Q3’23 ReportExpert Collections containing AI21 Labs
Expert Collections are analyst-curated lists that highlight the companies you need to know in the most important technology spaces.
AI21 Labs is included in 6 Expert Collections, including Unicorns- Billion Dollar Startups.
Unicorns- Billion Dollar Startups
1,270 items
Artificial Intelligence
10,014 items
Companies developing artificial intelligence solutions, including cross-industry applications, industry-specific products, and AI infrastructure solutions.
Digital Content & Synthetic Media
2,287 items
The Synthetic Media collection includes companies that use artificial intelligence to generate, edit, or enable digital content under all forms, including images, videos, audio, and text, among others.
AI 100
200 items
Generative AI 50
50 items
CB Insights' list of the 50 most promising private generative AI companies across the globe.
Generative AI
1,299 items
Companies working on generative AI applications and infrastructure.
AI21 Labs Patents
AI21 Labs has filed 19 patents.
The 3 most popular patent topics include:
- computational linguistics
- natural language processing
- tasks of natural language processing
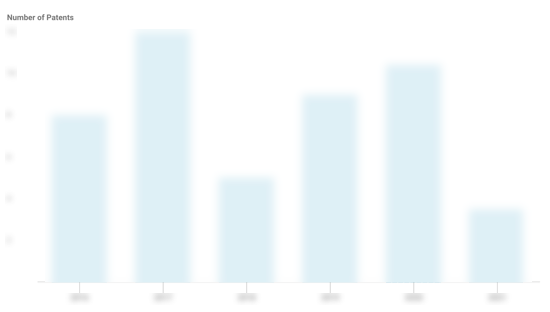
Application Date | Grant Date | Title | Related Topics | Status |
---|---|---|---|---|
5/11/2023 | 2/18/2025 | Natural language processing, Computational linguistics, Tasks of natural language processing, Semantics, Artificial intelligence applications | Grant |
Application Date | 5/11/2023 |
---|---|
Grant Date | 2/18/2025 |
Title | |
Related Topics | Natural language processing, Computational linguistics, Tasks of natural language processing, Semantics, Artificial intelligence applications |
Status | Grant |
Latest AI21 Labs News
Mar 25, 2025
Credit: Image generated by VentureBeat with StableDiffusion 3.5 Large Join our daily and weekly newsletters for the latest updates and exclusive content on industry-leading AI coverage. Learn More Transformer- based large language models (LLMs) are the foundation of the modern generative AI landscape. Transformers aren’t the only way to do gen AI, though. Over the course of the last year, Mamba, an approach that uses Structured State Space Models (SSM ), has also picked up adoption as an alternative approach from multiple vendors, including AI21 and AI silicon giant Nvidia. Nvidia first discussed the concept of Mamba-powered models in 2024 when it initially released the MambaVision research and some early models. This week, Nvidia is expanding on its initial effort with a series of updated MambaVision models available on Hugging Face . MambaVision, as the name implies, is a Mamba-based model family for computer vision and image recognition tasks. The promise of MambaVision for enterprise is that it could improve the efficiency and accuracy of vision operations, at potentially lower costs, thanks to lower computational requirements. What are SSMs and how do they compare to transformers? SSMs are a neural network architecture class that processes sequential data differently from traditional transformers. While transformers use attention mechanisms to process all tokens in relation to each other, SSMs model sequence data as a continuous dynamic system. Mamba is a specific SSM implementation developed to address the limitations of earlier SSM models. It introduces selective state space modelling that dynamically adapts to input data and hardware-aware design for efficient GPU utilization. Mamba aims to provide comparable performance to transformers on many tasks while using fewer computational resources Nvidia using hybrid architecture with MambaVision to revolutionize Computer Vision Traditional Vision Transformers (ViT) have dominated high-performance computer vision for the last several years, but at significant computational cost. Pure Mamba-based approaches, while more efficient, have struggled to match Transformer performance on complex vision tasks requiring global context understanding. MambaVision bridges this gap by adopting a hybrid approach. Nvidia’s MambaVision is a hybrid model that strategically combines Mamba’s efficiency with the Transformer’s modelling power. The architecture’s innovation lies in its redesigned Mamba formulation specifically engineered for visual feature modeling, augmented by strategic placement of self-attention blocks in the final layers to capture complex spatial dependencies. Unlike conventional vision models that rely exclusively on either attention mechanisms or convolutional approaches, MambaVision’s hierarchical architecture employs both paradigms simultaneously. The model processes visual information through sequential scan-based operations from Mamba while leveraging self-attention to model global context — effectively getting the best of both worlds. MambaVision now has 740 million parameters The new set of MambaVision models released on Huggi ng Face is available under the Nvidia Source Code License-NC, which is an open license. The initial variants of MambaVision released in 2024 include the T and T2 variants, which were trained on the ImageNet-1K library. The new models released this week include the L/L2 and L3 variants, which are scaled-up models. “Since the initial release, we’ve significantly enhanced MambaVision, scaling it up to an impressive 740 million parameters,” Ali Hatamizadeh, Senior Research Scientist at Nvidia wrote in a Hugging Face discussion post . “We’ve also expanded our training approach by utilizing the larger ImageNet-21K dataset and have introduced native support for higher resolutions, now handling images at 256 and 512 pixels compared to the original 224 pixels.” According to Nvidia, the improved scale in the new MambaVision models also improves performance. Independent AI consultant Alex Fazio explained to VentureBeat that the new MambaVision models’ training on larger datasets makes them much better at handling more diverse and complex tasks. He noted that the new models include high-resolution variants perfect for detailed image analysis. Fazio said that the lineup has also expanded with advanced configurations offering more flexibility and scalability for different workloads. “In terms of benchmarks, the 2025 models are expected to outperform the 2024 ones because they generalize better across larger datasets and tasks, Fazio said. Enterprise implications of MambaVision For enterprises building computer vision applications, MambaVision’s balance of performance and efficiency opens new possibilities Reduced inference costs: The improved throughput means lower GPU compute requirements for similar performance levels compared to Transformer-only models. Edge deployment potential: While still large, MambaVision’s architecture is more amenable to optimization for edge devices than pure Transformer approaches. Improved downstream task performance: The gains on complex tasks like object detection and segmentation translate directly to better performance for real-world applications like inventory management, quality control, and autonomous systems. Simplified deployment: NVIDIA has released MambaVision with Hugging Face integration, making implementation straightforward with just a few lines of code for both classification and feature extraction. What this means for enterprise AI strategy MambaVision represents an opportunity for enterprises to deploy more efficient computer vision systems that maintain high accuracy. The model’s strong performance means that it can potentially serve as a versatile foundation for multiple computer vision applications across industries. MambaVision is still somewhat of an early effort, but it does represent a glimpse into the future of computer vision models. MambaVision highlights how architectural innovation—not just scale—continues to drive meaningful improvements in AI capabilities. Understanding these architectural advances is becoming increasingly crucial for technical decision-makers to make informed AI deployment choices. Daily insights on business use cases with VB Daily If you want to impress your boss, VB Daily has you covered. We give you the inside scoop on what companies are doing with generative AI, from regulatory shifts to practical deployments, so you can share insights for maximum ROI. Subscribe Now
AI21 Labs Frequently Asked Questions (FAQ)
When was AI21 Labs founded?
AI21 Labs was founded in 2017.
Where is AI21 Labs's headquarters?
AI21 Labs's headquarters is located at 14 Leonardo da Vinci, Floor 4, Tel-Aviv.
What is AI21 Labs's latest funding round?
AI21 Labs's latest funding round is Series C - II.
How much did AI21 Labs raise?
AI21 Labs raised a total of $317M.
Who are the investors of AI21 Labs?
Investors of AI21 Labs include Ahren, Intel Capital, Comcast Ventures, Walden Catalyst Ventures, Pitango Venture Capital and 12 more.
Who are AI21 Labs's competitors?
Competitors of AI21 Labs include OpenAI, Anthropic, Together AI, LightOn, Writer and 7 more.
What products does AI21 Labs offer?
AI21 Labs's products include Jamba 1.6 and 1 more.
Who are AI21 Labs's customers?
Customers of AI21 Labs include Fnac and Educa Edtech.
Loading...
Compare AI21 Labs to Competitors
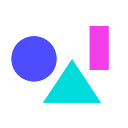
One AI specializes in generative artificial intelligence (AI) within the technology sector. The company offers services such as language analytics, customizable AI skills, and the processing of text, audio, and video data into structured, actionable insights. It primarily serves sectors such as customer service, e-commerce, media, healthcare, and government. It was founded in 2021 and is based in San Francisco, California.
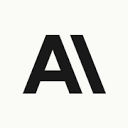
Anthropic operates a safety and research company focused on developing AI systems. The company's main offerings include Claude, an AI assistant for various tasks, and a suite of research initiatives aimed at AI safety and interpretability. Anthropic's research includes natural language processing, human feedback, reinforcement learning, and other areas. It was founded in 2021 and is based in San Francisco, California.
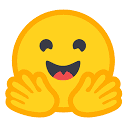
Hugging Face is an open-source machine learning platform that focuses on artificial intelligence within the technology sector. The company provides a space for the machine learning community to develop models, share datasets, host artificial intelligence (AI) applications, and offer enterprise solutions. It was founded in 2016 and is based in Paris, France.
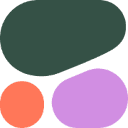
Cohere is an enterprise artificial intelligence (AI) platform that develops artificial intelligence solutions. The company offers AI models for text generation, document analysis, and assistant development, which can be integrated into enterprise workflows. Cohere's solutions serve various sectors including financial services, healthcare, manufacturing, energy, and the public sector. It was founded in 2019 and is based in Toronto, Canada.

Sakana AI focuses on developing artificial intelligence through nature-inspired foundation models within the research and development sector. The company's main offering includes creating a new kind of foundation model that draws inspiration from natural intelligence, designed to advance the field of AI. It was founded in 2023 and is based in Tokyo, Japan.

Goodfire AI specializes in artificial intelligent (AI) interpretability, focusing on creating tools for deploying safe and reliable generative AI models within the tech industry. The company provides infrastructure that enables developers to understand, edit, and debug AI models at scale, which facilitates the development of secure and dependable AI systems. Goodfire AI primarily serves sectors that require advanced AI model deployment and management, such as the tech industry and AI research organizations. It was founded in 2024 and is based in San Francisco, California.
Loading...