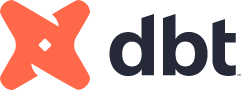
dbt Labs
Founded Year
2016Stage
Series D | AliveTotal Raised
$415.9MValuation
$0000Last Raised
$222M | 3 yrs agoRevenue
$0000Loading...
dbt Labs's Product Videos
_thumbnail.png?w=3840)
_(1)_thumbnail.png?w=3840)
ESPs containing dbt Labs
The ESP matrix leverages data and analyst insight to identify and rank leading companies in a given technology landscape.
Cloud database management systems provide a way for organizations to manage and control their data across cloud platforms and applications including collecting, storing, and securing data.
dbt Labs named as Outperformer among 10 other companies, including Cloudera, Databricks, and Yellowbrick Data.
dbt Labs's Products & Differentiators
dbt Cloud
dbt Cloud is a hosted solution for quickly and efficiently deploying trusted analytic code for downstream data use cases.
Loading...
Research containing dbt Labs
Get data-driven expert analysis from the CB Insights Intelligence Unit.
CB Insights Intelligence Analysts have mentioned dbt Labs in 1 CB Insights research brief, most recently on Oct 26, 2021.
Expert Collections containing dbt Labs
Expert Collections are analyst-curated lists that highlight the companies you need to know in the most important technology spaces.
dbt Labs is included in 2 Expert Collections, including Unicorns- Billion Dollar Startups.
Unicorns- Billion Dollar Startups
1,204 items
Artificial Intelligence
9,442 items
This collection includes startups selling AI SaaS, using AI algorithms to develop their core products, and those developing hardware to support AI workloads.
Latest dbt Labs News
Jan 20, 2023
January 21, 2023 - Advertisement - Data is a driving force for companies looking to make informed decisions. But gathering and interpreting that data can be a huge hurdle for many people. - Advertisement - When it comes to archiving, it’s not always as easy as buying a data set. For one, there must be efficient ways to interpret the data. Additionally, data teams need to be sure that the data they are actually getting is good data. With Big Data and analytics expected to be a huge market in 2023 (over $270 billion by some estimates), experts who specialize in interpreting and presenting data are vital to companies looking to use data to make informed decisions. do for - Advertisement - One such company is Bigeye, which was founded in 2019. I had a chance to speak with the company’s Kyle Kirwan to learn more about what they do, how they do it, and the importance of high-quality data. You can read the full interview below: Want to introduce yourself and your role in Bigeye? I’m the co-founder and CEO of BigEye. I started the company after leading a team at Uber that solved similar problems for their data team. In my career, I was one of the earliest analysts at Uber. There, I launched the company’s data catalog, DataBooks, as well as other tooling used by thousands of their internal data users. Bigeye is a Sequoia-backed startup working on data observables. you can reach me Twitter or LinkedIn. In just a few sentences, what does BigEye want to accomplish? We’re here to help data teams ensure that their stakeholders have access to high-quality data running on reliable data pipelines, without wasting time on drudgery manual efforts. Our data observability platform gives data engineers and scientists continuous visibility into the layout of their data pipelines, the performance of each pipeline, and the quality of the data running through them. This enables them to detect, understand, and resolve problems in their pipeline before they even reach analytics and machine learning applications. Image: Big Eye What inspired the creation of the company? I encountered a lot of data quality problems when I was a data scientist, and Egor (Egor Gryaznov, co-founder of Bigeye) had to fix a lot of data pipeline failures as a data engineer . That’s where our sympathy for the problem comes in. The actual company creation was inspired by conversations with people in data science and analytics who left Uber and missed the data testing, observation and incident management tools we had. We started to realize how important those tools had become and the fact that they didn’t exist outside of Uber and our peers at Airbnb and a few other companies. I started at Uber in 2013 as a data scientist, and watched many other data scientists, engineers, and analysts struggle as the company grew from ~100 data sets to over 100,000 data sets. It became difficult to know which data was accurate, reliable, up to date etc. and we often had breakdowns due to problems feeding data to analytics dashboards and machine learning models. I became a product manager for our data platform organization, reporting to the head of data, and led a team to develop internal tools to address those challenges at scale. Our tools are used each week by ~3000 people around the company to find data, see if it’s of high quality, and make sure our analysis and machine learning is working properly Used to be done. Bigeye is a way for me to continue that work, but in a way that serves a broader market, not just Uber. This is probably a loaded question, but how important is proactive data monitoring? It’s important, but it’s not the whole story! Monitoring is an important part of Observable, but it is like the meat in the middle of the sandwich. Instrumentation and alerting are two slices of bread. Without instrumentation, you have no signal from your systems to monitor, and without alerts to draw your attention to issues in the data pipeline, you have to remember to check your monitoring every day, which can get tedious. it happens. An overall data observable framework is critical for any business that relies on data (and it is most businesses). Let’s put it this way – if you don’t actively monitor the data, here’s what can go wrong: wasted team time lost trust in data In extreme cases loss of revenue running into lakhs Apart from data monitoring, does Bigeye provide any other services to the customers? Yes! Bigeye also automates the instrumentation process (we automatically track metadata like freshness and number of rows) about each table in a customer’s data warehouse. We automate alerts (we have developed an in-house anomaly detection system that outperforms simpler methods like ARIMA or Meta/Facebook’s Prophet library in real-world testing). Finally, we assist with root cause analysis of anomalies we detect (we automatically track data lineage and generate debugging queries that highlight specific rows of data affected by the anomaly). Walk me through the process – At a very basic level, how does Bigeye monitor data for anomalies and alert customers to issues? First, we connect to customer data sources such as analytics tools like Tableau or transformation tools like DBT. Next, we index all the data inside those sources so that it can be accessed by the customer, similar to a data catalog. Then we start automatically collecting table-level metadata about freshness, row count, and data lineage. We then use data profiling and our in-house recommendation algorithms to suggest more detailed column-level instrumentation (such as missing values, outliers, and correct data formats) that we think users should implement. And now that all those signals about their tables and columns are being tracked, we train an anomaly detection model (this happens in minutes) for each of the attributes that users associate with each attribute. Can alert you to problems. Users can receive those alerts in email, Slack, Microsoft Teams, etc. and based on how they react to them, receive model feedback that makes them more accurate over time, the more users our alerts answers to. Is your service for enterprise-level businesses or can SMBs and individuals benefit from Bigeye’s data monitoring? We typically work with organizations with 1,000 or more employees, and who have a total of 50+ people working on data engineering, data science, and analytics. But sometimes we work with smaller clients in industries like finance. Even a small organization can have a lot riding on its data, for example when using quantitative trading strategies. Where do you see the future of big data in the next 10 years? Now that infrastructure is easier to initially spin up and scale over time (thanks to companies like Snowflake, Databricks, Confluent, Astronomer, and DBT Labs), I see an increasing emphasis on data operations. This story unfolded very quickly inside Uber. Once we had all this great scalable data infrastructure that was open access within the company, managing it became a challenge. That’s where tools like Databook (our data catalog), Trust (our data testing system), and DQM (our data visualization system) come in. Companies are less and less excited about having data, and the focus is now on using data. Anything you would like to add before ending the interview? I love that data is woven more into society and general conversation. I hope that our results collectively demand more from organizations when it comes to how responsibly they treat the data that is being collectively generated.
dbt Labs Frequently Asked Questions (FAQ)
When was dbt Labs founded?
dbt Labs was founded in 2016.
Where is dbt Labs's headquarters?
dbt Labs's headquarters is located at 915 Spring Garden St, Philadelphia.
What is dbt Labs's latest funding round?
dbt Labs's latest funding round is Series D.
How much did dbt Labs raise?
dbt Labs raised a total of $415.9M.
Who are the investors of dbt Labs?
Investors of dbt Labs include Andreessen Horowitz, Amplify Partners, Sequoia Capital, Altimeter Capital, Coatue Management and 9 more.
Who are dbt Labs's competitors?
Competitors of dbt Labs include MarkLogic, Matillion, SingleStore, Databricks, Cockroach Labs and 13 more.
What products does dbt Labs offer?
dbt Labs's products include dbt Cloud.
Who are dbt Labs's customers?
Customers of dbt Labs include JetBlue, Monzo and Firefly Health.
Loading...
Compare dbt Labs to Competitors

Databricks provides a data platform that aims to simplify data integration and offers data analytic services. Databricks Lakehouse Platform serves corporations in various industries worldwide. It was founded in 2013 and is based in San Francisco, California.

Yellowbrick Data offers a modern data warehouse platform that breaks through the limitations of legacy and cloud-only alternatives. Based on a redesign of traditional analytic databases from the ground up, Yellowbrick Data Warehouse offers price/performance far beyond what alternatives can deliver, along with freedom to deploy in data centers/private clouds, public clouds, and both/hybrid.

Redis Labs is a private computer software company that provides a database management system marked as "NoSQL" as an open source software or as a service using cloud computing.

Crate.io is a scalable real-time database for the machine data era, such as information from the Internet of Things that stores massive amounts of data over the cloud and provides it via SQL connections.

Cloudera provides enterprise data management solutions that offer a unified platform for data, an enterprise data hub built on Apache Hadoop. It provides enterprises one place to store, process, and analyze all their data, empowering them to extend the value of existing investments while enabling fundamental new ways to derive value from their data.

Shakudo offers an end-to-end platform designed to help these teams turn their AI solutions into products more quickly. It does so by reducing their need for engineers, which are especially hard to come by these days.
Loading...