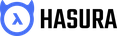
Hasura
Founded Year
2017Stage
Series C | AliveTotal Raised
$136.51MValuation
$0000Last Raised
$100M | 3 yrs agoMosaic Score The Mosaic Score is an algorithm that measures the overall financial health and market potential of private companies.
-46 points in the past 30 days
About Hasura
Hasura develops a data access layer for applications and artificial intelligence within the software development industry. The company offers a platform that aids in the creation, management, and evolution of application program interfaces (APIs), allowing data access for applications. Hasura's services are utilized by sectors that require data governance and integration with various databases. It was founded in 2017 and is based in San Francisco, California.
Loading...
Loading...
Expert Collections containing Hasura
Expert Collections are analyst-curated lists that highlight the companies you need to know in the most important technology spaces.
Hasura is included in 1 Expert Collection, including Unicorns- Billion Dollar Startups.
Unicorns- Billion Dollar Startups
1,270 items
Latest Hasura News
Mar 15, 2025
here I track enterprise software application development & data management. Follow Author Share getty Data has grades. Much like olive oil, flour, gasoline, beef and cotton, information is graded into various classes depending on its nature. We also classify data according to its state of managed structureness or unstructuredness, its state of deduplication, its mission-criticality, its speed of transport within a given network and its level of ratified veracity. All of which measures (and more) go some way towards enabling us to say whether we are dealing with high-quality data, or not. Why High-Quality Data Matters But if the “ingredients” in any given recipe are good enough to work (a cheap burger is still filling and anyone can fall asleep under low-grade cotton sheets), why does it matter whether we are using high-quality data or not? Business analysts would argue that refined information streams enable them to take more wide-ranging strategic business decisions; they would also propose that better customer/user experiences result from purified, ratified and amplified information provenance. Better data also helps firms more adroitly meet enhanced regulatory compliance targets, while it also helps reduce operational costs. But how do we keep tabs on whether an organization’s data is able to maintain its high-quality level… and mechanisms can we use to know when standards are slipping? Ken Stott , field CTO at real-time API engine and data access platform company Hasura says that the key to unlocking this value lies in implementing high-velocity feedback loops between data producers and data consumers. These feedback loops enable continuous communication and early issue detection, transforming how organizations manage their data assets. This proactive approach not only prevents problems at their source but creates a foundation for ongoing innovation and improvement. “Traditional data quality approaches rely on incident reporting systems and source-level checks. While effective for single domains, these methods break down at intersection points where data combines across boundaries,” explained Stott. “Take margin calculations - sales data and inventory data may each be accurate individually, yet quality issues emerge only when combined. When downstream teams discover these issues, their quality assessments often remain siloed - their insights rarely flow back upstream in a way source teams can effectively understand or act upon. This broken feedback loop perpetuates quality issues and slows organizational learning.” To enhance data quality, Stott’s recommendation to establish rapid feedback loops is said to enable a business to identify issues at the point of delivery and provide structured feedback for swift resolution. Understanding the typical organizational structure concerning data responsibilities is also important. Drawing from experience gained when working with its own customer base and further afield, the Hasura tech leader views three typical teams that share this duty. MORE FOR YOU Three Levels Of Data Responsibility Data domain owners: made up of data scientists and database administrators, network architects and software application developers (which might all be one person in reality) that manage data models, define quality rules and ensure data provisioning. Federated data teams: that oversee metadata standards, offer data discovery tools and manage data provisioning. Cross-domain data teams: that create “derived” data products (a term we have discussed and defined before here ), build reports and develop applications and models. “Cross-domain data users face unique challenges, as they often create the critical datasets that reach executive leadership and regulatory bodies,” detailed Stott, in a private press briefing this month. “Their need to compose and validate data across domains demands a modern approach built on: real-time validation with standardized metadata-driven feedback; centralized rule standards that maintain flexibility for local needs; integrated observability across the data lifecycle; and self-service composition capabilities for cross-domain teams.” Success here requires both organizational and technical foundations i.e. this vision only becomes a reality through lightweight additions to existing architectures: an extensible metadata-driven data access layer, automatable data quality rules and data-quality-as-a-service to assess composited datasets at the point of use. These enhancements create feedback loops without disrupting established data flows or requiring massive reorganization. “By adopting this approach, organizations can incrementally evolve their [data management and operational business] practices. Teams maintain their current workflows while gaining new collaboration capabilities. Clear ownership and accountability of data naturally emerges as feedback loops connect data producers and consumers. This foundation also paves the way for advanced capabilities like anomaly detection and sophisticated data profiling,” concluded Hasura’s Stott. “Ultimately, improving data quality isn’t just about technology, it’s about creating efficient processes that connect data producers and consumers.” By implementing automated feedback loops at the point of data delivery, the theory offered here suggests that organizations can significantly reduce the time and effort needed to identify and resolve data quality issues while preserving existing investments in data architecture and team structures. Data Diversity, Difficulty & Definiteness But is that the end of the data quality argument? Of course it isn’t and Stott had not set out to write the encyclopedia of data management with his essentially feedback-centric propositions. In his role as chief technology officer of AI model design for IoT company Aizip, Weier Wan has three key D(s) for us to embrace when it comes to data quality. “High-quality data only occurs when information channels are able to surface and evidence diversity,” saw Wan. “In this context I mean that data must be able to cover all scenarios so that the models based upon it can learn to generalize. It should also be a measure of data difficulty, so that means information resources that contain good amount of “difficult” examples, by which datasets that are inherently complex and not straightforward (i.e. requires more convoluted reasoning), ambiguous in nature and may often require specialized (perhaps graph and/or vector-based data analysis and management tools) to be able to extract value out of.” Wan also champions high-quality data to come out of information definiteness (we could say veracity to be conventionally aligned with the five Vs of big data , or we could simply say correctness which is the factor that Wan underlined) when it comes to working with data sources that will lead us to high quality data. “I’m talking about data definiteness or correctness where the business knows its information resources contain <1% of error, which is surprisingly not the case for almost all well-known public datasets." Of similar mind to Wan is Kjell Carlsson , head of AI strategy at Domino Data Lab. He says that enterprise leaders need to “dramatically rethink” data quality when it comes to AI. This is because efforts to clean, normalize and standardize data for one AI use case can easily remove signals and make it useless for others. “Standard methods of anonymizing data can alternately be unnecessary or ineffective depending on both the use case and the nature of the solution. Worse, most traditional approaches to data quality are not designed for the new types of unstructured data (documents, chats, emails, images, videos) used by AI solutions. Finally, what ‘quality’ data looks like usually only becomes apparent once an initial version of the solution is developed, rendering much of the data cleansing and engineering effort useless,” detailed Carlsson, in an online press briefing. For him, the answer to data quality for AI is to take a far more iterative and agile approach to quality control i.e. one that is integrated with and driven by the AI development and implementation lifecycle. Carlsson says that only by aligning data quality efforts to the actual needs of your AI use cases is the value of high quality data realized. But how do we ensure that sensitive data is protected during these cycles of development? Through governance, he says, that goes beyond data management, spans the activities of the AI lifecycle, and is tailored to the risks of the specific AI project. In a world of digitally encoded exactness across the information technology industry, a standardized and internationally codified measure of high-quality data is perhaps conspicuously absent. Given the number of databases, the diversity between different data management techniques and methodologies… and the now-neural level of interconnection points between applications, application programming interfaces and cloud datacenter backend services (let’s not even mention the encroaching universe of agentic AI functions), it’s not hard to see why there is room for debate here. Unlike good olive oil, high-quality data rarely comes from the first extra virgin “pressing”, but it does need Wagyu-level massaging like good beef and a fine-milled and refined approach like doppio-00 flour and well-aged cheese. It’s cheeseburger logic, basically.
Hasura Frequently Asked Questions (FAQ)
When was Hasura founded?
Hasura was founded in 2017.
Where is Hasura's headquarters?
Hasura's headquarters is located at 576 Folsom Street, San Francisco.
What is Hasura's latest funding round?
Hasura's latest funding round is Series C.
How much did Hasura raise?
Hasura raised a total of $136.51M.
Who are the investors of Hasura?
Investors of Hasura include Nexus Venture Partners, Vertex Ventures, Lightspeed Venture Partners, Greenoaks, STRIVE and 8 more.
Who are Hasura's competitors?
Competitors of Hasura include Quickwork, StepZen, Conektto, Prisma, Reltio and 7 more.
Loading...
Compare Hasura to Competitors
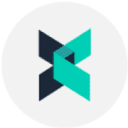
Crosscut is a French iPaaS company specializing in data integration and API management for cloud and on-premise environments. The platform enables organizations to exchange data between cloud applications and on-premise systems. Crosscut's services include data flow orchestration, real-time supervision, and integration of IoT, AI, big data, and RPA technologies. Crosscut was formerly known as Moskitos. It was founded in 2012 and is based in Paris, France.
Fliptin is a cloud-based API management platform. It offers a Cobuild-as-a-Service with pre-built integration capabilities. Its features include identity and security management, time-based tokenization, application containerization, and more. It was founded in 2016 and is based in Auckland Park, South Africa.
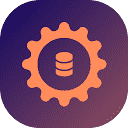
DreamFactory Software specializes in the automation of REST API generation. The company provides a platform that allows developers to create secure REST APIs from various databases and data sources without writing code. DreamFactory's solutions are utilized by sectors that require data integration and API management, such as healthcare, finance, and legal industries. It was founded in 2014 and is based in Campbell, California.
CorsairM360 specializes in API management solutions within the technology sector. The company offers a suite of products that facilitate the integration and management of APIs across multi-cloud, multi-tenant, and on-premise environments. Their main services include an API Gateway for seamless backend and frontend integration, a multi-technology Middleware SDK, and a Console for application configuration and operation. It was founded in 2020 and is based in Austin, Texas.

Meteor is a JavaScript framework that can be used for building applications across various platforms. It includes tools for developing and deploying web, mobile, and desktop applications with capabilities such as real-time updates and built-in authentication. It supports various front-end technologies. Meteor was formerly known as Honeycomb Guide. It was founded in 2011 and is based in San Francisco, California.
Nubo is a company focused on providing an edge computing platform for web application deployment and data management. The company offers a solution that allows developers to deploy TypeScript applications and manage globally distributed data with strong consistency, ensuring low latency and high performance for web applications. Nubo's platform is designed to be MySQL compatible and integrates with Prisma, offering built-in compliance features to meet various data privacy regulations. It was founded in 2020 and is based in London, England.
Loading...