Large language models (LLMs) trained on more text will generally be superior to an LLM with less. As a result, expect publishers with valuable text content to become a licensing battleground for LLM makers and for language acquisition costs (LAC) to become a real expense.
Updated December 4, 2023.
Google is said to pay $15B per year to be the default search engine on Apple devices.
These traffic acquisition costs (TAC) are in aggregate over $50B a year for Google — but the exclusivity gained is critical for Google to cement its search lead.
With the battle for large language model (LLM) supremacy underway, exclusive access to language/text will also become critical.
Big tech, all with generative AI ambitions, will look for publishers to become exclusive to their AI agents to achieve 2 objectives — improve their models and weaken others by depriving them of access.
So if language acquisition costs emerge, who should big tech lock up? We dig into some of the factors affecting LLMs and share 21 licensing targets below.
Is more text better for LLMs?
Yes, generally speaking, a large language model (LLM) that has access to more text information will be superior to one that doesn’t have the same access. This is because the performance of LLMs depends largely on the amount and quality of data they are trained on.
The more text data an LLM has access to, the better it can recognize patterns in language and make predictions about what words or phrases should come next.
In addition, having access to a wider range of text data can help LLMs to perform better on a variety of tasks. For example, if an LLM is trained on text data from many different sources, it may be better able to understand and generate language across a variety of domains and use cases — whether it be for commerce & intention or knowledge/research answers.
Of course, it is worth noting that simply having access to more text data isn’t always enough to make an LLM superior. The quality of the data is also important, as is the way that the LLM is trained and fine-tuned. Nonetheless, in general, having access to more text information is a key factor in the performance of large language models.
Who are the most attractive LLM licensing targets?
The choice of who to target from a licensing perspective will depend on what prompts the licensor is hoping to satisfy.
Large language models will be used primarily for two types of prompts, which can be boiled down to:
- Commerce & intention — This is people seeking answers to help inform buying decisions (e.g., what’s the best car? What’s the best hotel? What’s the best microwave? And so on)
- Knowledge/research — This is people seeking direct answers to questions. This could be about sports, science, politics, etc.
Of course, the 2 may be related. What begins as a research or knowledge prompt may evolve into a commerce prompt (or vice versa).
The licensing targets highlighted below cover everything from healthcare to software development to e-commerce to entertainment to stock investing and more.
Many are UGC (user-generated content) sites and many are publicly available, so restricting and licensing their content may require some changes.
The list also includes some surprising old names that you might not expect to see but which continue to tally up millions of visitors per month and which have a ton of historical text upon which to train LLMs.
With that, here are the 21 licensing targets — presented in no particular order — with a view into:
- Their monthly traffic
- Their current valuation
- Ownership status
- Similar companies
- Prompts they’d be useful for
1. Instructables (15M visits/month)
A platform for sharing step-by-step instructions for a wide range of DIY projects, from cooking to crafting to technology.
- Current status: Acquired
- Owner: Autodesk
- Type of prompt: Both
- Similar companies: Hackster.io
Want to see more research? Join a demo of the CB Insights platform.
If you’re already a customer, log in here.
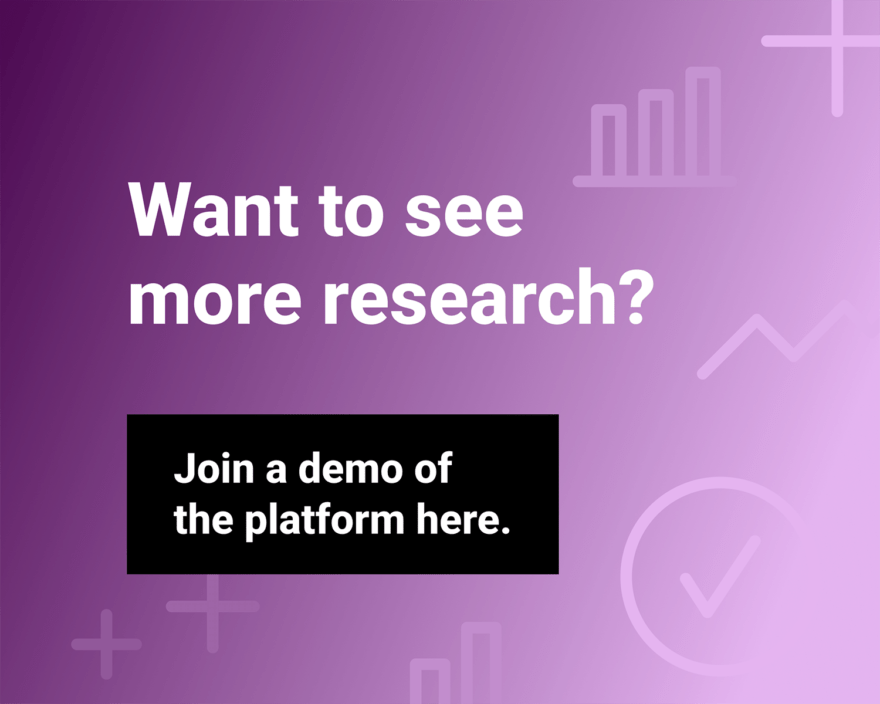