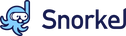
Snorkel AI
Founded Year
2019Stage
Incubator/Accelerator | AliveTotal Raised
$135MMosaic Score The Mosaic Score is an algorithm that measures the overall financial health and market potential of private companies.
+13 points in the past 30 days
About Snorkel AI
Snorkel AI specializes in data-centric artificial intelligence solutions for the enterprise domain. The company offers an AI data development platform that enables the development of AI applications by programmatically labeling and curating data, fine-tuning large language models, and building specialized AI models. It primarily serves sectors such as banking, healthcare, government, insurance, and telecom with its AI technology. The company was founded in 2019 and is based in Redwood City, California.
Loading...
Snorkel AI's Product Videos
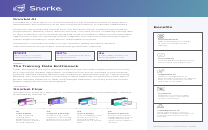
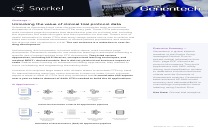
ESPs containing Snorkel AI
The ESP matrix leverages data and analyst insight to identify and rank leading companies in a given technology landscape.
The data annotation market provides services for labeling large volumes of data in preparation for training AI and ML models. This market comprises solutions for text, image, video, and audio annotation, with offerings ranging from fully managed human annotation services to AI-assisted automation platforms. Companies in this market serve enterprises across healthcare, automotive, retail, and techn…
Snorkel AI named as Leader among 15 other companies, including Scale, TELUS International, and Labelbox.
Snorkel AI's Products & Differentiators
Snorkel Flow
Enterprises often lack the resources of large AI developers but still need specialized AI models designed to work with their unique data and meet specific operational requirements. Snorkel Flow revolutionizes enterprise AI development by programmatically addressing the most significant bottleneck: data labeling and curation. Instead of relying on manual, time-intensive processes, Snorkel Flow allows users to label and curate data programmatically using rules, heuristics, and automation. It is 10–100x faster than traditional methods, producing higher-quality datasets in an iterative, adaptable, and auditable way. By shifting data labeling and curation from manual tasks to programmatic workflows, Snorkel Flow enables domain experts—like doctors, researchers, and legal professionals—to focus on meaningful, high-impact work.
Loading...
Research containing Snorkel AI
Get data-driven expert analysis from the CB Insights Intelligence Unit.
CB Insights Intelligence Analysts have mentioned Snorkel AI in 7 CB Insights research briefs, most recently on Oct 11, 2024.
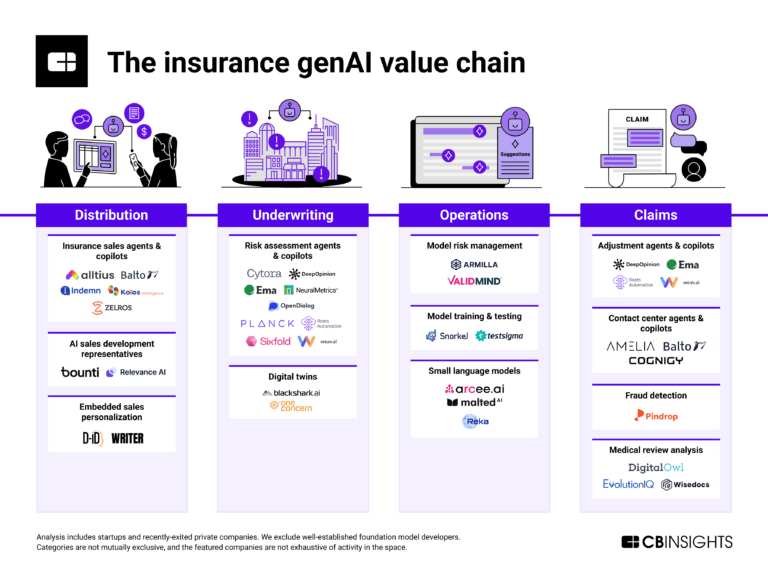
Oct 11, 2024
How genAI is reshaping the insurance value chain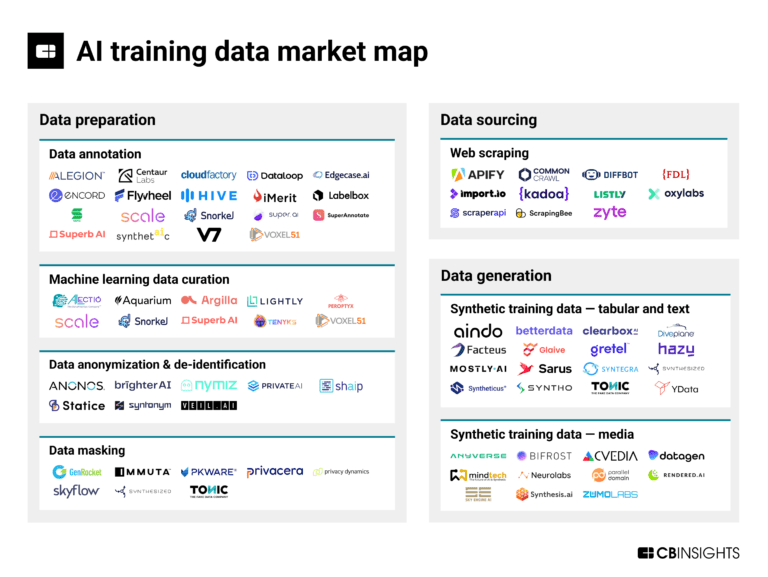
Feb 20, 2024
The AI training data market map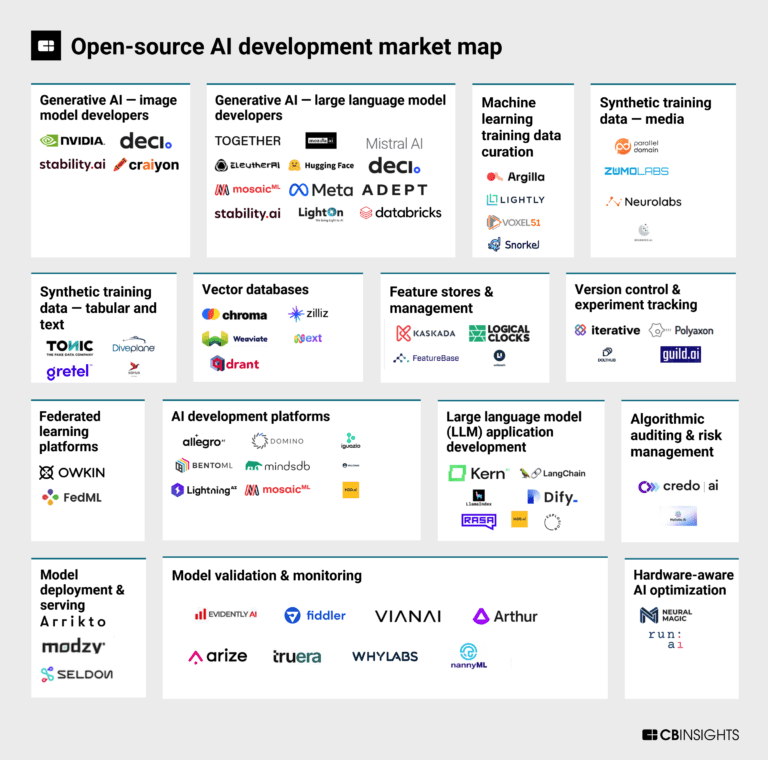
Oct 13, 2023
The open-source AI development market map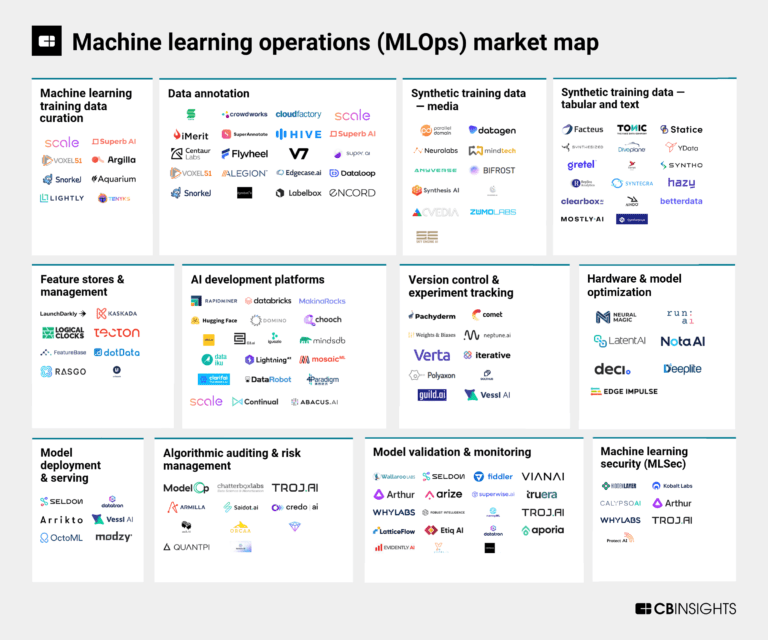
Sep 29, 2023
The machine learning operations (MLOps) market map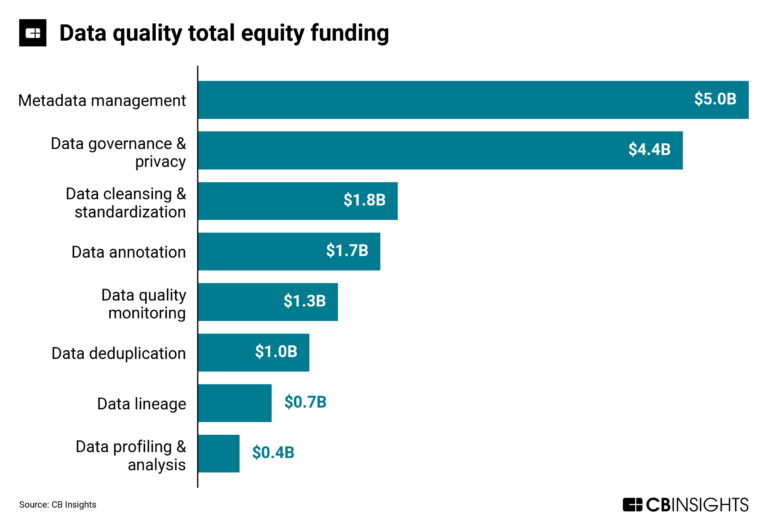
Jul 31, 2023
The data quality market mapExpert Collections containing Snorkel AI
Expert Collections are analyst-curated lists that highlight the companies you need to know in the most important technology spaces.
Snorkel AI is included in 4 Expert Collections, including Unicorns- Billion Dollar Startups.
Unicorns- Billion Dollar Startups
1,270 items
AI 100
100 items
Generative AI
1,299 items
Companies working on generative AI applications and infrastructure.
Artificial Intelligence
7,221 items
Snorkel AI Patents
Snorkel AI has filed 3 patents.
The 3 most popular patent topics include:
- artificial neural networks
- classification algorithms
- machine learning
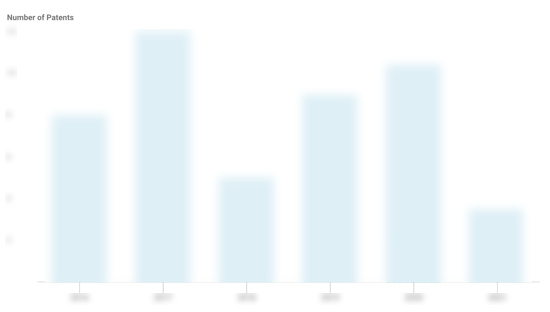
Application Date | Grant Date | Title | Related Topics | Status |
---|---|---|---|---|
6/26/2023 | Machine learning, Classification algorithms, Statistical classification, Artificial neural networks, Natural language processing | Application |
Application Date | 6/26/2023 |
---|---|
Grant Date | |
Title | |
Related Topics | Machine learning, Classification algorithms, Statistical classification, Artificial neural networks, Natural language processing |
Status | Application |
Latest Snorkel AI News
Feb 6, 2025
The DeepSeek Moment In the rapidly evolving world of AI, the arrival of DeepSeek-R1 marks a pivotal shift. We reached out to founders of five leading AI infrastructure companies in Greylock’s portfolio—Devvret Rishi (Predibase), Tuhin Srivastava (Baseten), Ankur Goyal (Braintrust), Jerry Liu (LlamaIndex), and Alex Ratner (Snorkel AI)—to get their take on DeepSeek and what it means for the future of open-source vs. closed models, AI infrastructure, and GenAI economics. Their insights reveal broad enthusiasm about the advancements DeepSeek brings, with some divergence in perspectives on its real-world impact. Open-Source vs. Closed Models: The Playing Field Has Leveled Historically, proprietary models like OpenAI’s were significantly ahead of open-source alternatives, often by 6-12 months. DeepSeek-R1 has fundamentally closed that gap, demonstrating performance on par with OpenAI’s latest offerings on key reasoning benchmarks, despite its smaller footprint. According to Rishi, “DeepSeek-R1 is a watershed moment for open-source AI. Historically, open-source models trailed proprietary models like OpenAI’s by 6-12 months. Now, DeepSeek-R1 has essentially closed that gap and is on par with OpenAI’s latest models on key reasoning benchmarks despite its smaller footprint.” He believes this is the inflection point where open-source begins to commoditize the model layer. Srivastava echoes this sentiment, stating, “DeepSeek as a moment changes everything. An open-source model is at the same level of quality as the SOTA closed-source models, which previously was not the case. Now with the cat out of the bag, all of the open-source models like Llama, Qwen, and Mistral will be soon to follow.” Goyal offers a more balanced perspective: “DeepSeek is the ‘LLaMa moment’ for O1-style (reasoning) models. In the very worst case, it means that the secret formula is out, and we’ll have a vibrant and competitive LLM market just like we did for GPT, Claude, and LLaMa-style models. In the best case, engineering teams will have a huge diversity of practical options, each with compute, cost, and performance trade-offs that enable them to solve a very wide variety of problems. In all cases, I think the world benefits from this progress.” Ratner echoes the above sentiments about open source and model diversity, focusing on the massive acceleration to enterprises specializing their own AI that this represents: “We’ve always viewed open source models as right behind- algorithms and model architectures rarely stay hidden for long. It was only a matter of time. However: it punctuates the exciting reality that enterprises will have a plethora of performant and cheap LLM options to then evaluate and specialize with their data and expertise, for their unique use cases. I expect DeepSeek to accelerate this trend of specialized enterprise AI massively.” AI Infrastructure and Developer Adoption: The Reinforcement Learning Revolution One of the most striking aspects of DeepSeek-R1 is its use of reinforcement learning (RL) to improve reasoning capabilities. While RL-based LLM optimization has been explored for years, DeepSeek is the first open-source model to successfully implement it at scale with measurable gains, using a technique called Generalized Reinforcement Learning with Policy Optimization (GRPO). Rishi sees this as a game-changer, explaining, “DeepSeek’s most impactful contribution is proving that pure reinforcement learning can bootstrap advanced reasoning capabilities, as seen with their R1-Zero model.” However, he also notes a major gap: “Most ML teams have never trained reasoning models, and today’s AI tooling isn’t built to support this new paradigm.” Srivastava believes that DeepSeek marks an inflection point for AI infrastructure: “With DeepSeek, the moat of ‘we trained the biggest and best model, so we’re keeping it closed’ is gone. Now, you have a situation where frontier model-level quality is available in a model you completely control.” Ratner adds, “We are seeing the ‘AlphaGo moment’ for constrained, verifiable domains (e.g. math, basic coding- things that are simple and well defined to check for correctness), showing the power of LLMs + RL, and the rapid diffusion of algorithmic advancements.” However, he stresses that the next steps will involve expanding this success to more complex and less verifiable domains—a much longer and more challenging journey, involving much more human ingenuity and input. New Applications: AI Reasoning at Scale DeepSeek’s improved reasoning capabilities unlock a new wave of applications. Rishi highlights several emerging possibilities, including: Autonomous AI agents that refine their decision-making over time. Highly specialized planning systems in industries like finance, logistics, and healthcare. Enterprise AI assistants that dynamically adapt to user needs beyond rigid RAG-based solutions. AI-powered software engineering tools that can self-debug and optimize code based on performance feedback. Liu expands on this by discussing the implications for GPU demand and agentic applications: “DeepSeek does not mean that there will be less demand for GPU compute; on the contrary, I’m quite excited because I think it will significantly accelerate the demand and adoption of agentic applications. One of the core issues with building any agent that ‘actually works’ is reliability, speed, and cost—robust agent applications that can e2e automate knowledge workflows require reasoning loops that repeatedly make LLM inference calls. The more general/capable the agent is, the more reasoning loops it requires. While the O1/O3 series of models have impressive reasoning capabilities, they are way too cost prohibitive to use for more general agents. Faster/cheaper models incentivize the development of more general agents that can solve more tasks, which in turn leads to greater demand and adoption across more people and teams.” Srivastava emphasizes, “There are big implications from DeepSeek and the subsequent models that will follow for highly regulated industries. Companies that have strict data compliance requirements will be able to more freely experiment and innovate knowing that they can completely control how data is used and where it’s sent.” Ratner underscores that data remains the real edge: “R1-style progress relies on strong pre/post-training in the relevant domains and rigorous evaluation data before the RL magic kicks in.” He further explains, “Many things in GenAI today effectively reduce to really high-quality, domain-specific labeling, including reinforcement learning. DeepSeek’s results reiterate the point that if you have a good way to label (i.e. a “reward function”), you can do magic; but in most domains, it’s not so easy to get the data or label it.” Economics of GenAI: The Cost Equation Changes DeepSeek accelerates the trend toward cheaper, more efficient inference and post-training, significantly altering the economics of GenAI deployment. Rishi points to Jevon’s Paradox: “As LLMs become cheaper and more efficient, enterprises won’t just replace proprietary APIs with open-source models—they’ll use AI even more, fine-tuning and deploying multiple domain-specific models instead of relying on a single general-purpose one.” Srivastava emphasizes the financial impact, stating, “A reasoning model like R1 will be as much as 7x cheaper than using an OpenAI or Anthropic. Having a model with those economics unlocks many use cases that previously may not have been financially viable or attractive for most enterprises, which are yet to come meaningfully online with Gen AI. Ratner adds, “As the generators become more powerful and commoditized—it’s all about labeling! Whether it’s RLHF, heuristic methods like DeepSeek-R1’s reward functions, or advanced hybrid approaches, defining the acceptance function to match the distribution we want is the logical next step.” DeepSeek is undeniably a milestone in the AI industry, marking the first time an open-source model has reached true competitive parity with proprietary alternatives. Rishi and Srivastava see it as a fundamental shift, ushering in a new era of AI development where companies can fully control high-performing models while benefiting from the economic advantages of open-source. Goyal, however, remains skeptical of its real-world adoption so far, viewing it more as a pricing lever against incumbents than an immediate replacement. Ratner reinforces that while DeepSeek represents meaningful progress, much of the future of AI hinges on high-quality, domain-specific data and labeling. He concludes, “Whether you see it as a paradigm shift or just another step in the AI arms race, its impact on the industry is undeniable.” From Idea to Iconic.
Snorkel AI Frequently Asked Questions (FAQ)
When was Snorkel AI founded?
Snorkel AI was founded in 2019.
Where is Snorkel AI's headquarters?
Snorkel AI's headquarters is located at 55 Perry Street, Redwood City.
What is Snorkel AI's latest funding round?
Snorkel AI's latest funding round is Incubator/Accelerator.
How much did Snorkel AI raise?
Snorkel AI raised a total of $135M.
Who are the investors of Snorkel AI?
Investors of Snorkel AI include Google Cloud Next, QBE Ventures, Greylock Partners, Google Ventures, Lightspeed Venture Partners and 10 more.
Who are Snorkel AI's competitors?
Competitors of Snorkel AI include Centaur Labs, Ydata, Encord, Scale, Accrete and 7 more.
What products does Snorkel AI offer?
Snorkel AI's products include Snorkel Flow.
Who are Snorkel AI's customers?
Customers of Snorkel AI include Experian, Wayfair and Bank of America.
Loading...
Compare Snorkel AI to Competitors
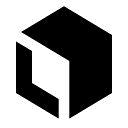
Labelbox provides services and software for artificial intelligence (AI) data management and model evaluation within the artificial intelligence and machine learning sectors. The company offers managed labeling services, a platform for building data factories, and a network for hiring experienced AI trainers. Labelbox serves AI teams and organizations seeking to improve their model training and evaluation. It was founded in 2018 and is based in San Francisco, California.

Scale provides data labeling, model training, and curation services for artificial intelligence (AI) applications, along with a generative AI platform that uses enterprise data to improve AI models. Scale serves the technology sector, government agencies, and the automotive industry. Scale was formerly known as Scale Labs. It was founded in 2016 and is based in San Francisco, California.
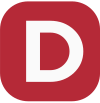
Dbrain focuses on document recognition and conversion technologies within the financial services sector. The company provides products that enable the extraction and processing of data from various types of documents, including passports and invoices, for KYC and anti-fraud purposes. Dbrain's offerings are used by banks, insurance companies, and government agencies for operational tasks and compliance. It is based in Moscow, Russian Federation.
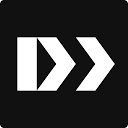
Datasaur specializes in NLP data labeling and LLM development platforms. It offers a suite of tools for customizable data annotation, quality control management, and automation to enhance the efficiency of NLP and LLM projects. Datasaur's products are designed to meet the complex needs of industries such as legal, healthcare, financial, media, e-commerce, and government. It was founded in 2019 and is based in Livermore, California.
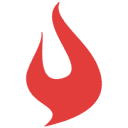
iMerit provides data annotation solutions for enterprise artificial intelligence (AI). The company offers a data labeling platform known as Ango Hub that includes tools for image, video, text, and audio annotation, as well as sentiment analysis, lidar annotation, content moderation, product categorization, and image segmentation. It primarily serves industries such as autonomous vehicles, medical AI, agriculture, financial services, and technology. It was founded in 2012 and is based in San Jose, California.
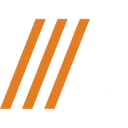
Alegion focuses on data annotation and collection services and operates within the artificial intelligence and machine learning industry. The company offers services such as data collection, data annotation, and quality control, aimed at transforming unstructured data into high-quality, model-ready training data. Alegion primarily serves sectors such as healthcare, hospitality, insurance, manufacturing, retail, security, software, and sports. It was founded in 2012 and is based in Austin, Texas.
Loading...