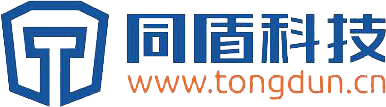
Tongdun Technology
Founded Year
2013Stage
Series E | AliveTotal Raised
$361.44MValuation
$0000Last Raised
$115M | 6 yrs agoMosaic Score The Mosaic Score is an algorithm that measures the overall financial health and market potential of private companies.
-50 points in the past 30 days
About Tongdun Technology
Tongdun Technology specializes in intelligent risk management and anti-fraud services within the financial and internet sectors. The company offers solutions that integrate artificial intelligence to provide efficient risk control and credit risk assessment for various industries. Tongdun Technology primarily serves financial institutions and internet firms with its intelligent risk management and anti-fraud solutions. Tongdun Technology was formerly known as FraudMetrix. It was founded in 2013 and is based in Hangzhou, Zhejiang .
Loading...
Loading...
Expert Collections containing Tongdun Technology
Expert Collections are analyst-curated lists that highlight the companies you need to know in the most important technology spaces.
Tongdun Technology is included in 6 Expert Collections, including Unicorns- Billion Dollar Startups.
Unicorns- Billion Dollar Startups
1,270 items
Regtech
1,453 items
Technology that addresses regulatory challenges and facilitates the delivery of compliance requirements. Regulatory technology helps companies and regulators address challenges ranging from compliance (e.g. AML/KYC) automation and improved risk management.
Payments
3,136 items
Companies in this collection provide technology that enables consumers and businesses to pay, collect, automate, and settle transfers of currency, both online and at the physical point-of-sale.
Artificial Intelligence
9,687 items
Companies developing artificial intelligence solutions, including cross-industry applications, industry-specific products, and AI infrastructure solutions.
Cybersecurity
10,544 items
These companies protect organizations from digital threats.
Fintech
13,699 items
Excludes US-based companies
Latest Tongdun Technology News
Jul 10, 2023
Spatial Data Makes AI Crop Yield Predictions Better Share Explore by topic Topics Support IEEE Spectrum IEEE Spectrum is the flagship publication of the IEEE — the world’s largest professional organization devoted to engineering and applied sciences. Our articles, podcasts, and infographics inform our readers about developments in technology, engineering, and science. A not-for-profit organization, IEEE is the world's largest technical professional organization dedicated to advancing technology for the benefit of humanity. IEEE websites place cookies on your device to give you the best user experience. By using our websites, you agree to the placement of these cookies. To learn more, read our Privacy Policy. Saving articles to read later requires an IEEE Spectrum account The Institute content is only available for members Downloading full PDF issues is exclusive for IEEE Members Access to Spectrum's Digital Edition is exclusive for IEEE Members Following topics is a feature exclusive for IEEE Members Adding your response to an article requires an IEEE Spectrum account Create an account to access more content and features on IEEE Spectrum, including the ability to save articles to read later, download Spectrum Collections, and participate in conversations with readers and editors. For more exclusive content and features, consider Joining IEEE . Join the world’s largest professional organization devoted to engineering and applied sciences and get access to all of Spectrum’s articles, archives, PDF downloads, and other benefits. Learn more → Special offer: Join IEEE now for 2023 and save 50%! IEEE Members receive 12 print issues of IEEE Spectrum and enjoy PDF downloads, full access to our archive with thousands of in-depth articles, and other exclusive content and features. Join IEEE today for 2023 and save 50%! Climate change has made the need for accurate predictions more urgent than ever 3 min read Even as agricultural techniques have improved, climate change and higher demand have resulted in less wiggle room for predicting crop yields. BanksPhotos/Getty Images This article is part of our exclusive IEEE Journal Watch series in partnership with IEEE Xplore. Researchers from Zhejiang University and risk management company Tongdun Technology, both based in Hangzhou, China, have improved crop yield predictions using deep learning techniques. It’s a promising method that can account for the way crop yield is affected by the location of farmland, and can help produce more accurate predictions for farmers and policymakers. Predicting crop yield is an important part of agriculture that has historically consisted of tracking factors like weather and soil conditions. Making accurate predictions gives farmers an edge when making financial decisions for their businesses and helps governments avoid catastrophes like famine. Climate change and increasing food production have made accurate predictions more important than ever as there’s less room for error. Climate change is increasing the risk of low crop yields in multiple regions , which could cause a global crisis. Many of the variables used to predict crop yield—like the climate, soil quality, and crop management methods—are still the same, but modeling techniques have become more sophisticated in recent years. Deep learning techniques not only can calculate how variables like precipitation and temperature affect crop yield, but also how they affect each other. The benefits of increased rain, for example, can be canceled out by extremely hot temperatures. The way variables interact can lead to different results than looking at each variable independently. In their study, the researchers used a recurrent neural network , which is a deep learning technique that tracks the relationships of different variables through time, to help capture “complex temporal dependencies” affecting crop yield. Variables relating to crop yield that are affected by time include temperature, sunlight, and precipitation, said Chao Wu , a researcher at Zhejiang University and one of the paper’s authors. Wu said these factors “change over time, interact with each other in complex ways, and their impact on crop yield is usually cumulative.” This technique is also able to infer the effect of variables that are difficult to quantify, such as steady improvements in breeding and agricultural cultivation techniques, Wu said. As a result, their model benefited from capturing larger trends that stretched beyond a single year. The researchers also wanted to incorporate spatial information, like information about the proximity between two regions of farmland to help determine whether their crop yields are likely to be similar. To do so, researchers combined their recurrent neural network with a graph neural network representing geographic distance to determine how predictions for particular locations would be impacted by the area around them. In other words, the researchers could include information about adjacent regions for each area of farmland, and help the model learn from relationships across time and space. The researchers tested their new method on US soybean yield data published by the National Agricultural Statistics Service . They input climate data including precipitation, sunlight, and vapor pressure; soil data like electrical conductivity, acidity, and soil composition; and management data like the percentage of fields planted. The model was trained on soybean yield data between 1980 and 2013, and tested using data from 2015 to 2017. Compared with existing models, the proposed method performed significantly better than models trained using non-deep learning methods, and better than other deep learning models that did not take spatial relationships into account. In their future work, the researchers want to make the training data more dynamic and add security features to the model training process. Currently, the model is trained on data that has been aggregated, which doesn’t allow the possibility of keeping proprietary data private. This could be a problem if data like crop yields and farm management practices is seen by competitors and used to gain an unfair advantage in the marketplace, Wu said. Agricultural data like farm location and crop yields could also make farmers vulnerable as the target of scams and theft. The possibility of data disclosure could also deter participation, decreasing the amount of data available to train on and negatively impacting the accuracy of trained models. Researchers hope to use a federated learning approach to train future crop yield models, which would allow the training to update a global model while keeping different sources of data isolated from each other.
Tongdun Technology Frequently Asked Questions (FAQ)
When was Tongdun Technology founded?
Tongdun Technology was founded in 2013.
Where is Tongdun Technology's headquarters?
Tongdun Technology's headquarters is located at Room 207, Building 18, Future Science and Technology City (Haichuang Park), No. 998 Wenyi West Road, Hangzhou.
What is Tongdun Technology's latest funding round?
Tongdun Technology's latest funding round is Series E.
How much did Tongdun Technology raise?
Tongdun Technology raised a total of $361.44M.
Who are the investors of Tongdun Technology?
Investors of Tongdun Technology include GF Securities, AVIC Capital, ZheShang Venture Capital, Cinda SinoRock Investment Management, GGV Capital and 14 more.
Who are Tongdun Technology's competitors?
Competitors of Tongdun Technology include NetGuardians, 4Paradigm, DataVisor, Fraud.net, Trusfort and 7 more.
Loading...
Compare Tongdun Technology to Competitors
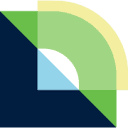
DataVisor focuses on fraud and risk management within the financial technology sector. It offers an AI-powered SaaS platform that provides enterprise-level fraud prevention and AML compliance solutions. The company primarily serves financial institutions and large organizations, offering a suite of tools to combat various types of fraud and financial crimes. It was founded in 2013 and is based in Mountain View, California.

Trusfort provides business security services, proposing the concept of “human-oriented business security”. Trusfort uses business security technology, artificial intelligence to create information security and offer a whole lifecycle business security protection solution based on scenarios to help customers build a safe, intelligent, and trustworthy business system. The company was founded in 2015 and is based in Beijing, China.

Shumei Technology specializes in AI-driven online business risk control solutions within the technology sector. The company offers a suite of intelligent detection services for text, images, audio, video, webpages, and documents to identify and mitigate various content risks. Shumei Technology primarily serves sectors that require robust online risk management, such as e-commerce, social media, and financial services. It was founded in 2015 and is based in Beijing, China.
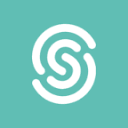
SEON focuses on fraud prevention and anti-money laundering (AML) compliance. The company offers products that include digital footprint analysis, device intelligence, and artificial intelligence (AI) and machine learning to detect and prevent fraudulent activities in real-time. SEON's solutions address various use cases such as account takeover, bonus abuse, and transaction monitoring. It was founded in 2017 and is based in Budapest, Hungary.

Ahi Fintech uses AI and human expert Integration systems to help clients make predictions and manage risk in their industries.
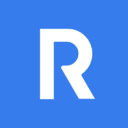
RealAI provides security detection and security reinforcement services. It is a developer of artificial intelligence solutions, including an unsupervised learning algorithm, an interpretability algorithm, decision-making solutions, anti-fraud and anomaly detection, and more. It caters to healthcare, energy, environment, education, banking, and services industries. The company was founded in 2018 and is based in Haidian, China.
Loading...